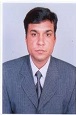
Raj Kumar
Sant Longowal Institute of Engineering and Technology, India
Title: Hilbert Hunag tranform based EMG signal analysis for forearm movements and classification using Ann
Biography
Biography: Raj Kumar
Abstract
EMG (Electromyogram) signal contains wealth information about muscle function which is widely used in clinical and engineering application to investigate muscle activity. EMG signals are acquired by surface electrodes which are placed on the targeted muscle set. In order to use the EMG signal as a diagnosis signal or a control signal, a feature is often extracted before performing analysis or classification stage. This study would provide a method for myoelectric control of the movement of forearm prosthetic limbs and human-machine interface for applications in different tasks as hand close, hand open, supination, pronation, flextion, extension. EMG signals has been recorded from BIOPAC MP 100 c System, the forearm muscles of subjects for six movement set mentioned above. The healthy subject aged between 23-30 years have been used for this purpose. The recording was done for six different motions using two active surface electrodes were placed on the skin surface covering the ‘Flexor Carpi Ulnaris’ and ‘Brachioradialis’ muscle in the forearm in differential mode placed with 20 millimeter apart. The single surface electrode was placed on the unconcerned muscle for the reference purpose. Time-Frequency domain features were calculated to extract the information from the signals. ANN has been used to classify between these different movements of forearm which gives accuracy of 85.56% and 86.26% for channel 1and channel 2 (left and right hand) at IMF-1 level with 10 number of hidden layers which are trained by scaled conjugate gradient method for supervised learning in MATLAB.